Generative vs Predictive AI: Key Differences & Real-World Applications
Generative vs Predictive AI: A Practical Comparison
Generative vs Predictive AI: Key Differences & Real-World Applications
Generative vs Predictive AI
Exploring the differences between Generative and Predictive AI, this article delves into their unique traits and real-world applications.
Real "AI Buzz" | AI Updates | Blogs | Education
Predictive AI
Generative AI
Generative AI is a type of AI that can create new content, such as text, images, music, and code. It does this by learning from existing data and then generating new data that is similar to the training data. For example, a generative AI model can be trained on a dataset of ad copy examples and then used to generate new creative and effective ad copies.
The basic difference is that predictive AI outputs predictions and forecasts, while generative AI outputs new content. Here are a few examples across various domains:
- Natural Language Processing (NLP): Predictive NLP models can categorize text into predefined classes (e.g., spam vs. not spam), while generative NLP models can create new text based on a given prompt (e.g., a social media post or product description).
- Image processing: Predictive image processing models, such as convolutional neural networks (CNNs), can classify images into predefined labels (e.g., identify different products on a grocery store shelf). On the other hand, generative models like diffusion models can create new images that are not present in the training data (e.g., virtual models for advertising campaigns).
- Drug discovery: Predictive drug discovery models can forecast whether a new compound is likely to be toxic or have potential as a new drug treatment. Generative drug discovery models can create new molecular structures with desired properties, such as higher efficacy or lower toxicity.
How Do Predictive vs. Generative AI Algorithms Work
Subheading
Predictive AI analyzes past data to forecast future events. It relies on supervised learning, needing labeled data with input-output pairs. The model learns relationships from this data and uses it to predict new outcomes. Predictive AI employs various techniques like linear regression and deep learning for tasks such as predicting sales or customer behavior.
The predictive AI algorithms can be used to predict a wide range of variables, including continuous variables (e.g., sales volume) and binary variables (e.g., whether a customer will churn). They can be based on basic machine learning models like linear regression, logistic regression, decision trees, and random forests. In some cases, deep learning algorithms and reinforcement learning demonstrate exceptional performance for predictive AI tasks thanks to their ability to learn complex patterns in data.
Let’s say a healthcare provider wants to use predictive AI to identify patients at risk of heart disease. They may use historical data from their previous patients to see how different features, such as patients’ demographic data, health conditions, and treatments, were associated with heart disease. Machine learning models can spot unexpected patterns and provide quite accurate predictions about which patients are more likely to develop heart disease. Healthcare providers can then use these predictions to develop personalized prevention plans.
In contrast to predictive AI, generative AI models are typically trained using unsupervised or semi-supervised learning algorithms. This means that they do not require large amounts of labeled data. Unsupervised learning algorithms learn from unlabeled data, while semi-supervised learning algorithms learn from a combination of unlabeled data and a small amount of labeled data.
Basically, most of the current generative AI models are built by masking part of the training data and then training the model to recover the masked data.
Another common type of generative AI model are diffusion models for image and video generation and editing. These models are built by first adding noise to the image and then training the neural network to remove noise.
Both LLMs and diffusion models can achieve outstanding performance when trained on sufficiently large amounts of unlabeled data. However, to improve results for specific use cases, developers often fine-tune generative models on small amounts of labeled data.
Strengths and Weaknesses
When it comes to predictive AI, here are the key benefits of using this technology:
- High accuracy: Predictive AI models can be trained to achieve very high accuracy for many tasks, such as product recommendation, fraud detection, and risk assessment.
- Automation: Predictive AI can automate many tasks and free up human workers to focus on more strategic and creative work.
However, this type of AI comes with its challenges, such as for example:
- Labeled data requirement: Predictive AI models require labeled data, which can be expensive and time-consuming to collect.
- High bar for success: Predictive AI applications need to be highly accurate to be successful. This can be difficult to achieve, especially for complex tasks.
- Model maintenance: Predictive AI models need to be regularly retrained on new data in order to maintain their accuracy. This can be a challenge for companies with limited resources.
Generative AI algorithms have their own strengths points:
- Increased productivity and efficiency: Generative AI can make the process of content creation, code writing, image creation, and designing much faster. This can save businesses a significant amount of time and money.
- Creativity: Generative AI can generate new and innovative ideas that humans may not have thought of. This can help businesses to develop new products and services, and to improve their existing products and services.
However, as a very new technology, it has a number of challenges to take into account, including:
- Lack of reliability: Generative AI applications tend to be highly unreliable. They may produce false or misleading information, and will usually require a human in the loop for any customer-facing applications.
- Reliance on pretrained models: Businesses typically need to rely on externally created pretrained models for generative AI applications. This can limit their control over the model and its output.
- Copyright and intellectual property issues: There are copyright and intellectual property concerns surrounding the use of generative AI models. For example, it is unclear who owns the copyright to the content generated by a generative AI model that was trained on copyrighted data.
These strengths and weaknesses largely determine the key application areas for generative AI and predictive AI. Let’s take a closer look.
Read More
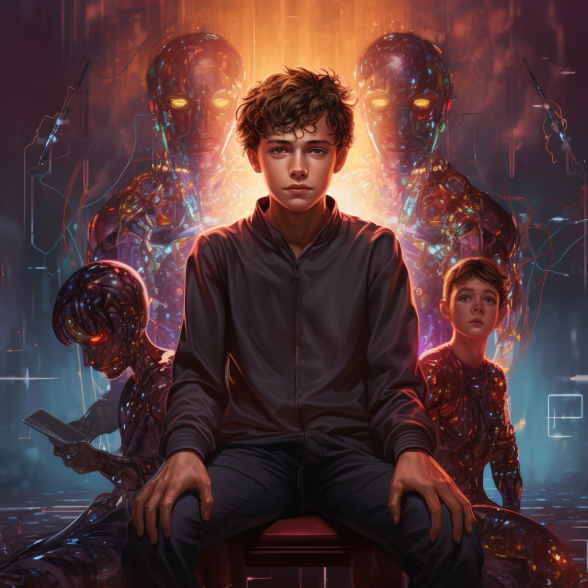
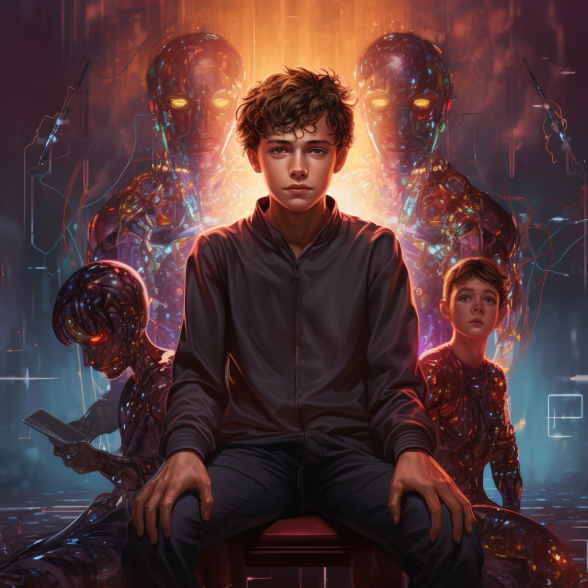
Leave a Reply