What is the UnSupervised Learning in Machine Learning- InDepth Explanation
What is the UnSupervised Learning in Machine Learning- InDepth Explanation
What is the UnSupervised Learning in Machine Learning- InDepth Explanation
Table of Contents
UnSupervised Learning in ML
UnSupervised Learning
Unsupervised learning is the training of a machine using information that is neither classified nor labeled and allowing the algorithm to act on that information without guidance. Here the task of the machine is to group unsorted information according to similarities, patterns, and differences without any prior training of data.
Unlike supervised learning, no teacher is provided that means no training will be given to the machine. Therefore the machine is restricted to find the hidden structure in unlabeled data by itself. For instance, suppose it is given an image having both dogs and cats which it has never seen.
Real "AI Buzz" | AI Updates | Blogs | Education
Thus the machine has no idea about the features of dogs and cats so we can’t categorize it as ‘dogs and cats ‘. But it can categorize them according to their similarities, patterns, and differences, i.e., we can easily categorize the above picture into two parts. The first may contain all pics having dogs in them and the second part may contain all pics having cats in them. Here you didn’t learn anything before, which means no training data or examples. It allows the model to work on its own to discover patterns and information that was previously undetected. It mainly deals with unlabelled data.
Unsupervised learning is classified into two categories of algorithms
- Clustering: A clustering problem is where you want to discover the inherent groupings in the data, such as grouping customers by purchasing behavior.
- Association: An association rule learning problem is where you want to discover rules that describe large portions of your data, such as people that buy X also tend to buy Y.
What is Unsupervised Learning?
Why use Unsupervised Learning?
Below are some main reasons which describe the importance of Unsupervised Learning: o Unsupervised learning is helpful for finding useful insights from the data. o Unsupervised learning is much similar as a human learns to think by their own experiences, which makes it closer to the real AI. o Unsupervised learning works on unlabeled and uncategorized data which make unsupervised learning more important. 99 o In real-world, we do not always have input data with the corresponding output so to solve such cases, we need unsupervised learning.
How Unsupervised Learning Works
Working of unsupervised learning can be understood by the below diagram:
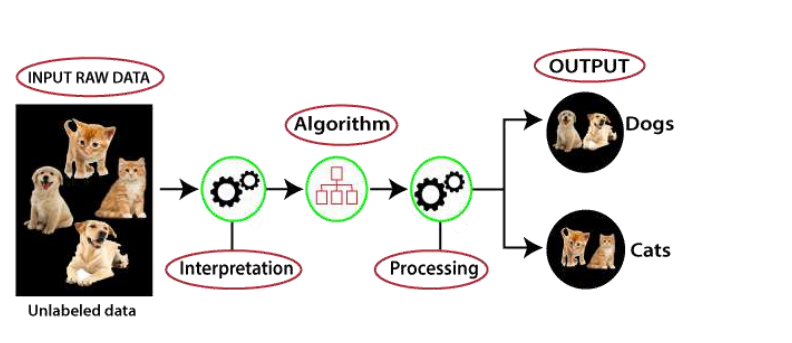
Here, we have taken an unlabeled input data, which means it is not categorized and corresponding outputs are also not given. Now, this unlabeled input data is fed to the machine learning model in order to train it. Firstly, it will interpret the raw data to find the hidden patterns from the data and then will apply suitable algorithms such as k-means clustering, Decision tree, etc. Once it applies the suitable algorithm, the algorithm divides the data objects into groups according to the similarities and difference between the objects
Types of Unsupervised Machine learning Algorithms
#1. Clustering
Clustering is a method of grouping the objects into clusters such that objects with most similarities remains into a group and has less or no similarities with the objects of another group. Cluster analysis finds the commonalities between the data objects and categorizes them as per the presence and absence of those commonalities.
#2. Association
An association rule is an unsupervised learning method which is used for finding the relationships between variables in the large database. It determines the set of items that occurs together in the dataset. Association rule makes marketing strategy more effective. Such as people who buy X item (suppose a bread) are also tend to purchase Y (Butter/Jam) item. A typical example of Association rule is Market Basket Analysis.
Unsupervised Learning algorithms:
Below is the list of some popular unsupervised learning algorithms:
o K-means clustering
o KNN (k-nearest neighbors)
o Hierarchal clustering
o Anomaly detection
o Neural Networks
o Principle Component Analysis
o Independent Component Analysis
o Apriori algorithm
o Singular value decomposition
Advantages of unsupervised learning:
- Unsupervised learning is used for more complex tasks as compared to supervised learning because, in unsupervised learning, we don’t have labeled input data.
- Unsupervised learning is preferable as it is easy to get unlabeled data in comparison to labeled data
Disadvantages of unsupervised learning
- Unsupervised learning is intrinsically more difficult than supervised learning as it does not have corresponding output.
- The result of the unsupervised learning algorithm might be less accurate as input data is not labeled, and algorithms do not know the exact output in advance.
Read More
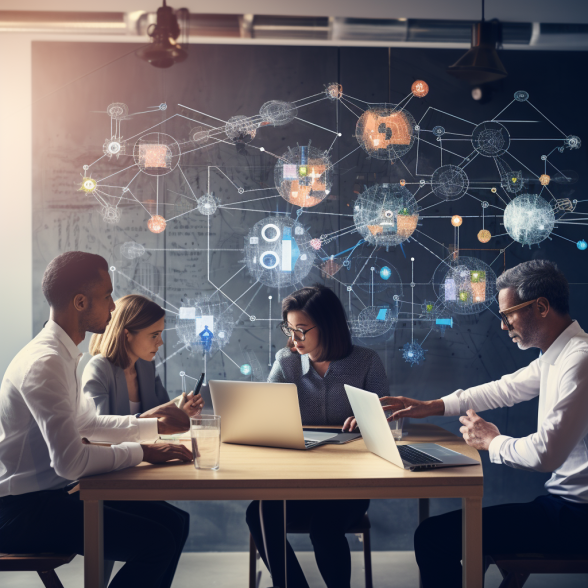
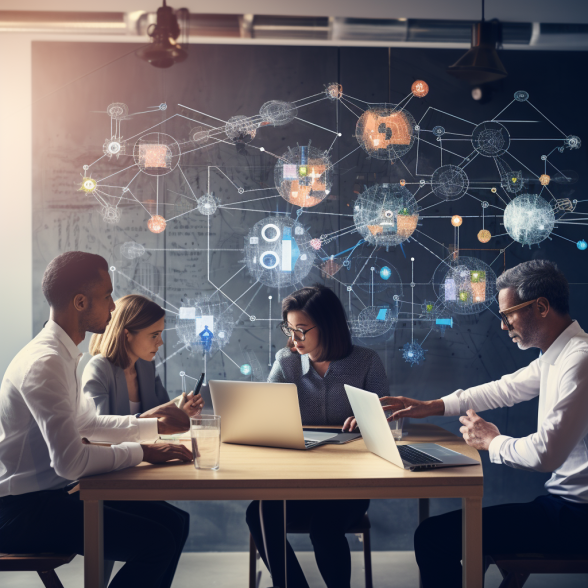
Leave a Reply