Recent advances in machine learning: the significance of AlphaGo- Some Interesting Facts
Recent advances in machine learning: the significance of AlphaGo
Recent advances in machine learning: the significance of AlphaGo- Some Interesting Facts
the significance of AlphaGo
AlphaGo
Progress in AI has frequently been marked by the ability of computer systems to play – and beat humans at – different games.
In the 1950s and 1960s, Arthur Samuel, a researcher at IBM, wrote a machine learning program that could play checkers. Samuel’s program determined its next move by using a search-tree to compute possible moves and evaluating the board position which resulted from each option. The machine built up an understanding of ‘good’ and ‘bad’ moves via repeated games, and used this to conduct its assessment of the state of the board.
Although it never achieved expert-level play – it was characterised as better than average – Samuel’s system marked a major milestone in the history of AI for its ability to learn strategies by playing against itself.
Real "AI Buzz" | AI Updates | Blogs | Education
In 1997, Deep Blue became the first computer chess-playing system to beat a reigning world chess champion, with its victory over Garry Kasparov receiving significant attention. Rather than relying on a revolutionary new algorithmic approach to game-playing, Deep Blue exploited the increased computing power available in the 1990s to perform large-scale searches of potential moves – it could reportedly process over 200 million moves per second – then pick the best one.
Then in 2011, IBM’s Watson was pitted against human players in the US quiz show Jeopardy, and beat two of the show’s champions, Brad Rutter and Ken Jennings.
The research that underpinned these developments sought to create rule-based systems, which encoded human knowledge about how to play the game and what moves to use in different situations. Armed with this knowledge, the computer could then use advanced search or decision-tree methods to select an appropriate response for a particular configuration of pieces. Essentially, these machines made use of increasing computing power to perform complex searches in order to select their next move.
Although successful in achieving certain tasks, this approach to replicating human intelligence was limited in its scalability and transferability: a chess-playing system could not play chequers, and a system relying on these types of rules could not be scaled to more challenging or intuitive games, such as the ancient Chinese game of Go.
The game Go originated in China over 2500 years ago. It is a game with relatively simple rules – players place stones on a board, and aim to cordon off empty space to create their territory, or to capture the stones of their opponent – but it is incredibly complex, due to the huge number of potential moves. Successful Go players therefore rely on intuition or instinct to play the game, rather than a rigid set of instructions.
Creating a computer which could win at Go was seen, until recently, as an uncompleted Grand Challenge in artificial intelligence.
In 2016, Google DeepMind’s AlphaGo system changed this. Traditional search-tree methods would not be able to process the incredibly large number of potential moves in Go. Researchers at DeepMind therefore followed a different approach: using stochastic searches and deep neural networks, they trained AlphaGo on 30 million moves from games played by humans. To further enhance its abilities, they then used reinforcement learning to allow AlphaGo to learn from thousands of games it played against itself.
This learning was put to the test in 2016, in a series of matches against Lee Sedol, who has been acknowledged as the world’s top Go player for over a decade. AlphaGo played five games against Lee Sedol; it won four of them.
These victories demonstrated the ability of machine learning to tackle hugely complex tasks, and in doing so to produce solutions which humans may not have considered; pivotal moves played by AlphaGo had only a 1 in 10,000 chance of being played by a human. These were considered to be highly surprising – even beautiful – by Go experts. The AlphaGo / Lee Sedol match therefore provided a further milestone in the development of machine learning, and the history of pitching humans against machines in games to test intelligence.
Read More
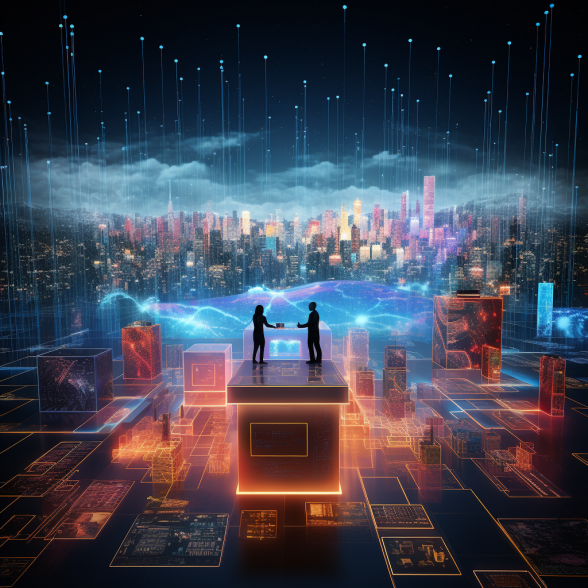
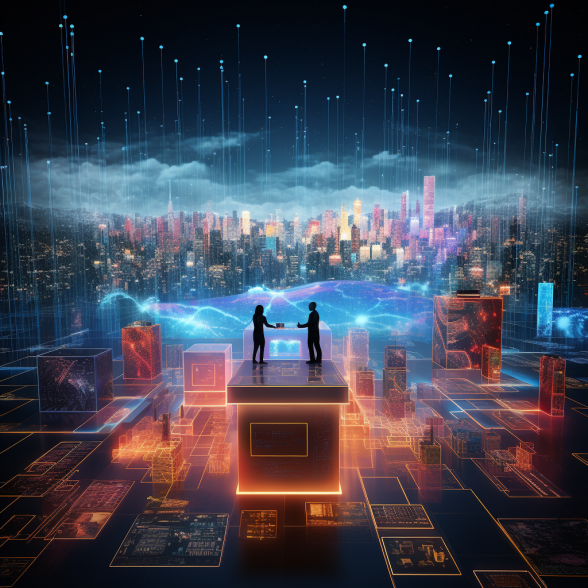
Leave a Reply